tl;dr - You’re not behind now, but 2025 is the year to place your bets:
Most GTM organizations are still experimenting with AI. Only a minority have systematic plans, despite promising early impact.
The most mature use cases for AI in GTM are content creation and research. Analytics and agents are more nascent. Invest carefully.
AI spend is surging, but GTM teams get a fraction of the overall budget. Most teams should spend on application-layer solutions and avoid building in-house capabilities directly on LLMs.
Marc Benioff and others are saying the quiet part out loud: AI is coming for information-based human labor. 2025 likely isn’t the year we slash headcount, but outsourced services like content production, SEO and data management should be in your crosshairs.
Read on for the full story.
—
I asked a question in the headline. That means it’s time to invoke Betteridge’s Law which states that any headline ending in a question mark can be answered with no. So…
No, you’re not behind. Yet.
However, it’s hard not to feel behind when Marc Benioff is reporting that Salesforce is now the “largest supplier of digital labor […] all powered by these autonomous AI agents” in their Q3 earnings call. Not to mention that there are at least 374 AI SDR companies and it’s impossible to scroll LinkedIn for more than 10 seconds without a post either about AI or generated by AI (or both).
Clearly things are moving fast. And unlike some other supposed revolutionary technologies, AI is actually transformational because it solves real problems. You need to pay attention and make some bets in 2025 or you really will be left behind.
We’re all finalizing our budgets for next year. It’s a good time to take stock of where most GTM orgs actually are on the road to AI adoption, where they’re heading next and how they’re paying for it.
What’s the state of AI adoption in GTM today?
Everyone certainly believes in a bright future for AI in GTM. According to Gartner, GTM organizations expect generative AI to increase sales productivity by more than 25% and revenue by more than 10%.1
With apologies to William Gibson, some of that future is here, it’s just not evenly distributed.2
And with Salesforce, Agentforce, we're not just imagining this future, we're already delivering it. And you still know that in the last week of the quarter, Agentforce went into production. We delivered 200 deals and our pipeline is incredible for future transactions. We can talk about that with you on the call, but we've never seen anything like it. We don't know how to characterize it.
— Marc Benioff, improving CRM’s stock price during Q3 earnings
So while Salesforce is delivering the future (one with fewer human workers—more on that later), the rest of us are still trying to figure it out.
Craig Rosenberg and Mark Gustaferro at Scale Venture Partners recently published an AI GTM “flash survey” of 63 GTM leaders. It’s a small sample size. It’s also likely that the GTM leaders who respond to a VC survey are reasonably close to the cutting edge of tech adoption. We can reasonably assume this represents the higher-end of real-world adoption.
A majority of respondents in the survey (33/63, 52%) are using AI ad-hoc without formal adoption. Without knowing the exact question, I’d say this sounds like employees using ChatGPT, Anthropic, etc on their own initiative for one-off tasks like drafting emails and basic research.
Only two orgs reported that they’ve “significantly overhauled” their function using AI. If you combine this with folks reporting they’re piloting or planning to use AI team-wide, you get just under 30% of respondents with even the concepts of a plan. If you’re not there yet, neither are most other people.
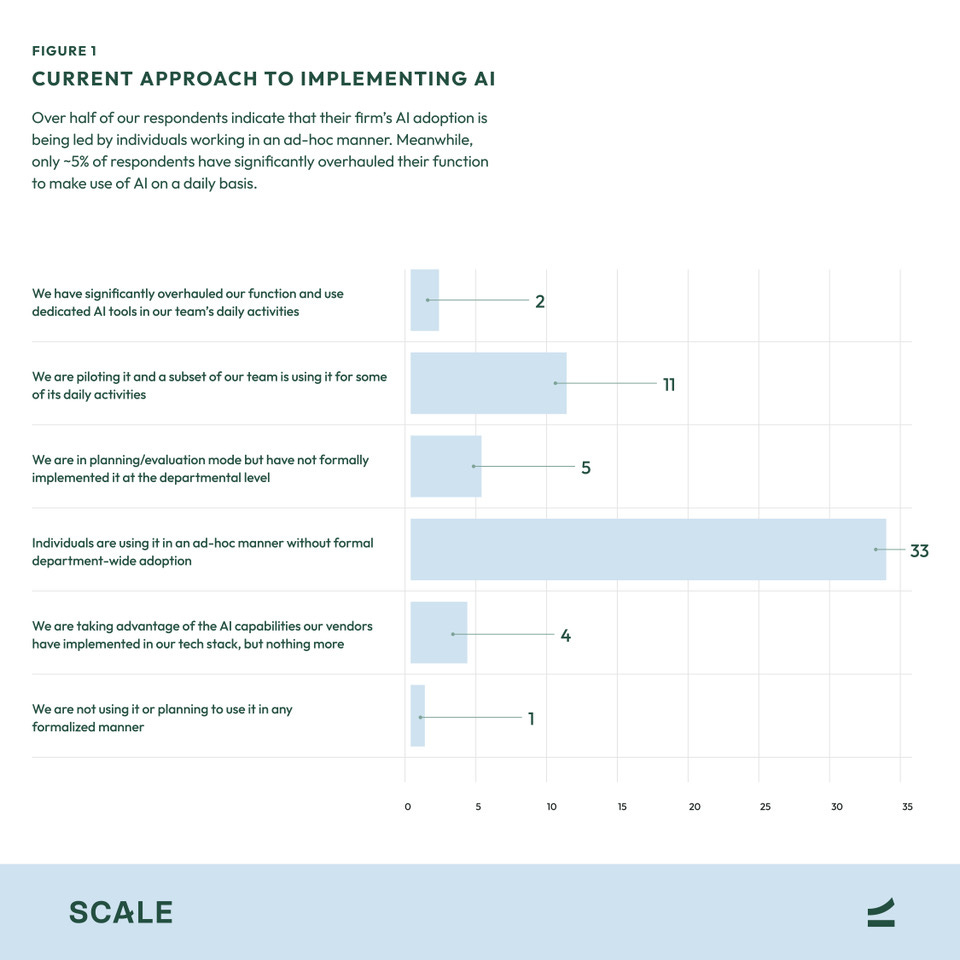
Even at these low levels of formal adoption, there are promising signs that AI makes a difference. More than half of respondents in the Scale survey reported at least a moderate impact from AI, with 17% reporting a strong or very strong impact.
Long story short, your team is most likely already using AI. In 2025, you should figure out how to apply it more systematically to get better results. That leads us to the next question: Apply it to what?
What are GTM teams actually doing with AI?
Scale isn’t the only venture firm in the AI survey game. Menlo Ventures recently published their 2024 report on The State of Generative AI in the Enterprise (they did it in 2023 as well).3
This report surveyed 600 decision-makers at companies with 50+ employees. It also isn’t GTM-specific, so it helps put things in perspective. These are the use cases that will compete with you for AI budgets. Broadly, the current killer app for Generative AI is writing code, followed by support chatbots as a distant second.
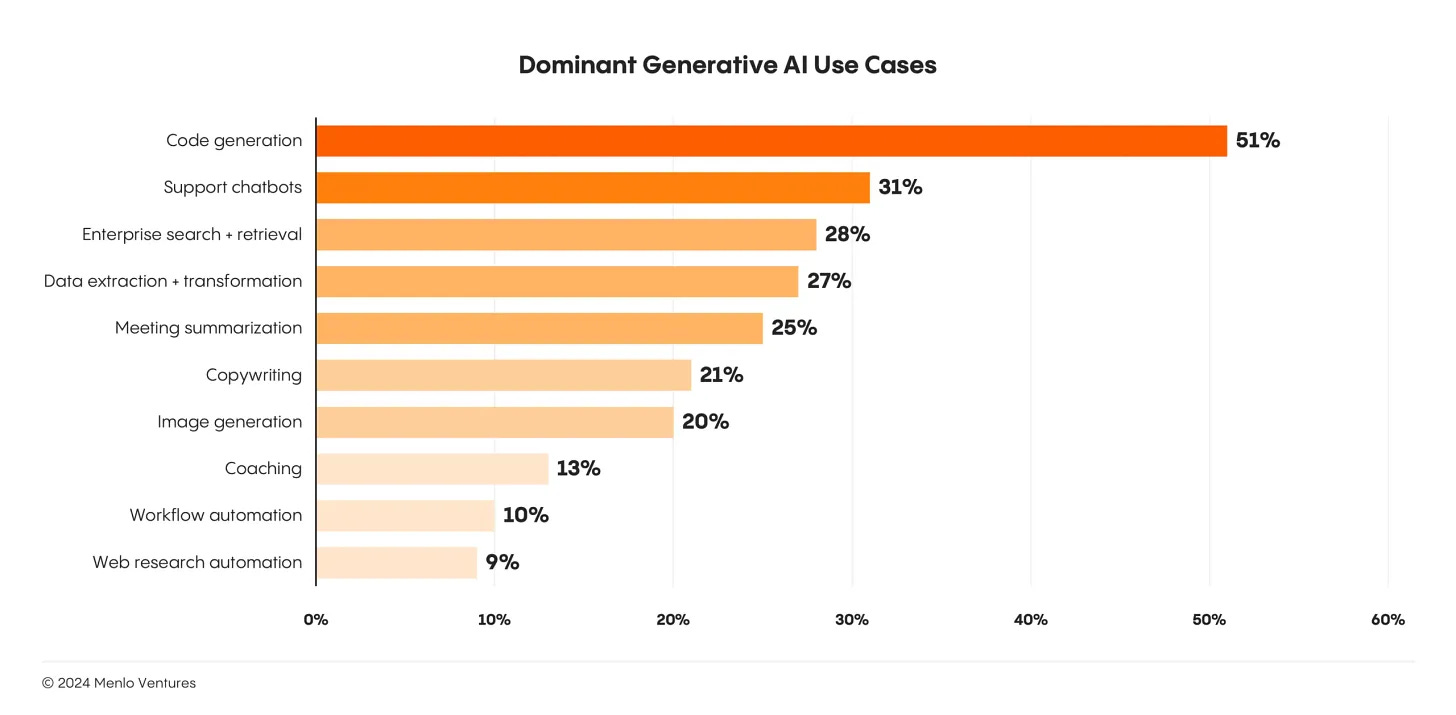
As you go a little farther down the list, you start to see use cases that are at least tangentially related to GTM: meeting summarization, copywriting, coaching, workflows and web research. You could also make an argument that search and retrieval would heavily involve CRM data as a source.
Scale’s survey, on the other hand, highlights specific GTM use cases:
While these don’t perfectly align to Scale’s use cases, I see four main themes:
Generation - Creating fairly general content like ad creative, blog posts and sales collateral along with more tailored content for specific comms out to targets. This can save a ton of time, but beware the uncanny valley.
Research - Gathering unstructured data from internal and external sources and synthesizing it into useful information for sales pitches, training or data enrichment.4
Analytics - Reviewing structured and unstructured data for trends and insights as well as to make predictions.
Agents - Using AI in a way that lets it reason and take specific actions to accomplish a goal in the real world (e.g. a CS bot that can handle a multi-step support request). This is what Benioff is so excited about and what the next generation of “co-pilots” will be able to do.
Generation is obviously where the current AI hype started and research is a natural outgrowth of how large language models can synthesize unstructured data. The analytics and agent use cases, however, are farther behind.
This isn’t surprising. General-purpose LLMs aren’t great at quantitative analysis. This is why OpenAI is investing in specialized models like o1 that build reasoning capabilities on top of LLMs. Right now these models are pricey and best applied sparingly. But, I wouldn’t be surprised to see them rapidly get cheaper and get incorporated more fully into RevOps and analytics stacks in 2025.
If you’re thinking about AI for analytics, make sure you really know what you want. Are you just making it easy for humans to query a data warehouse with natural language or are you actually expecting an intelligence that can proactively identify important information for you? The former works much better than the latter right now.
Agents that are capable of taking action either autonomously or on behalf of a human are, as you can tell from Benioff’s Agentforce hype, the next big thing. There’s still a lot to do here to makes these safe, trustworthy and effective. I think we’ll hear a ton about this in 2025, but you should be cautious about letting AI take the wheel.
What about budgets?
Enterprises are certainly spending on AI. Menlo Ventures reports that AI spending is up to nearly $14B in 2024 (+6x y/y)5. Two thirds of that spend is going towards the infrastructure layer, which is not where GTM teams should be investing.
About 25% of that overall spend is going towards departmental or horizontal AI. This (along with vertical AI) is the “application layer” in VC-speak. While this is interesting from a macro standpoint, it’s not super helpful as you think about the budget that might be available to your GTM org.
The Menlo Ventures survey says that 8% and 7% of enterprise AI spend is being allocated to Sales and Marketing, respectively (compared to 22% for IT and 19% for product and engineering). So, it may be reasonable to think your GTM team should be consuming 15-20% of your org’s AI budget (if such a thing exists).
Most of that budget should be allocated to the burgeoning number of application-layer companies that help GTM teams succeed. If you find your team getting in the weeds and building directly on LLMs, you should probably question that spend. You really should outsource the challenge of keeping up with the pace of AI change to someone else.
Which budget? Software, services or FTEs?
Many of us have historically budgeted with separate line items for software (e.g. CRM), outsourced services (e.g. consultants) and FTEs (e.g. AEs, BDRS, etc). AI is blurring those lines. The 2024 State of OpenCloud Report from Battery Ventures includes this telling market size slide:
They believe AI has a $4T (trillion with a T!) TAM because it can take on software, services and human labor all at once.
The big companies are starting to say the quiet part out loud: AI is coming for every kind of information-based human labor. Microsoft finally did more than just hint at it, while Salesforce is basically shouting it from the rooftops. Here’s the larger context from Benioff’s opening remarks on last week’s earnings call:
[…] for the last 25 years at Salesforce, and we've been helping companies to manage and share their information […] but now we've really created a whole new market, a new TAM, a TAM that is so much bigger and so much more exciting than the data management market, it's hard to get our head completely around.
This is the market for digital labor. And Salesforce has become right out of the gate here, the largest supplier of digital labor and this is just the beginning and it's all powered by these autonomous AI agents. All of you know that. This is fundamentally reshaping how businesses operate. It's fundamentally reshaping how we operate our business and how we think about the industry itself and how you're thinking about the industry, how we're thinking about Salesforce.
And with Salesforce, Agentforce, we're not just imagining this future, we're already delivering it. And you still know that in the last week of the quarter, Agentforce went into production. We delivered 200 deals and our pipeline is incredible for future transactions. We can talk about that with you on the call, but we've never seen anything like it. We don't know how to characterize it.
This is really a moment where productivity is no longer tied to workforce growth, but through this intelligent technology that can be scaled without limits.
There’s a big question here about the blurring line between software and labor—that’s a discussion for another time.
Ultimately, I don’t think 2025 is the year large swathes of headcount budget get reallocated to AI. I do think it’s the right time to ask if there are ways to replace services with AI. Content creation and SEO are probably the lowest-hanging fruit, followed by outsourced data quality management (i.e. BPO companies doing enrichment or CRM cleanup). You may have an opportunity to get much better bang for your buck there with less management overhead.
You’re not behind, but you will be
Betteridge remains undefeated. No, you’re not behind. But if you’re not already making meaningful investments in AI, you’re definitely not ahead. If you’re not making moves in 2025, you’ll fall behind the teams that do.
2025 should be the year that you make some rational bets on how AI can help your GTM team. If you’re not sure what to do, bet on the most mature use cases like research and content generation and find budget by reducing services.
Some of these bets won’t work out. The ones that do will likely set you up for transformational change in the coming years. Start now.
I’m not sure how these sales leaders think they’ll do that because I haven’t read the full report. I’m not made of analyst money.
“The future is already here, it’s just not evenly distributed” is usually attributed to author William Gibson. He may not have actually said it. He did, however, coin “cyberspace”.
The list of authors includes “with Claude Sonnet 3.5”. Not a bad way to indicate you used AI to write something.
Gradient Works is an example of this. AI Researcher does data enrichment from web content and Market Map finds customer lookalikes. That data then gets operationalized through account assignment.
Menlo Ventures explicitly excludes chips from this number, the costs of which are driving capex through the roof at big tech companies building foundation models.